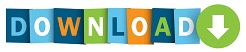
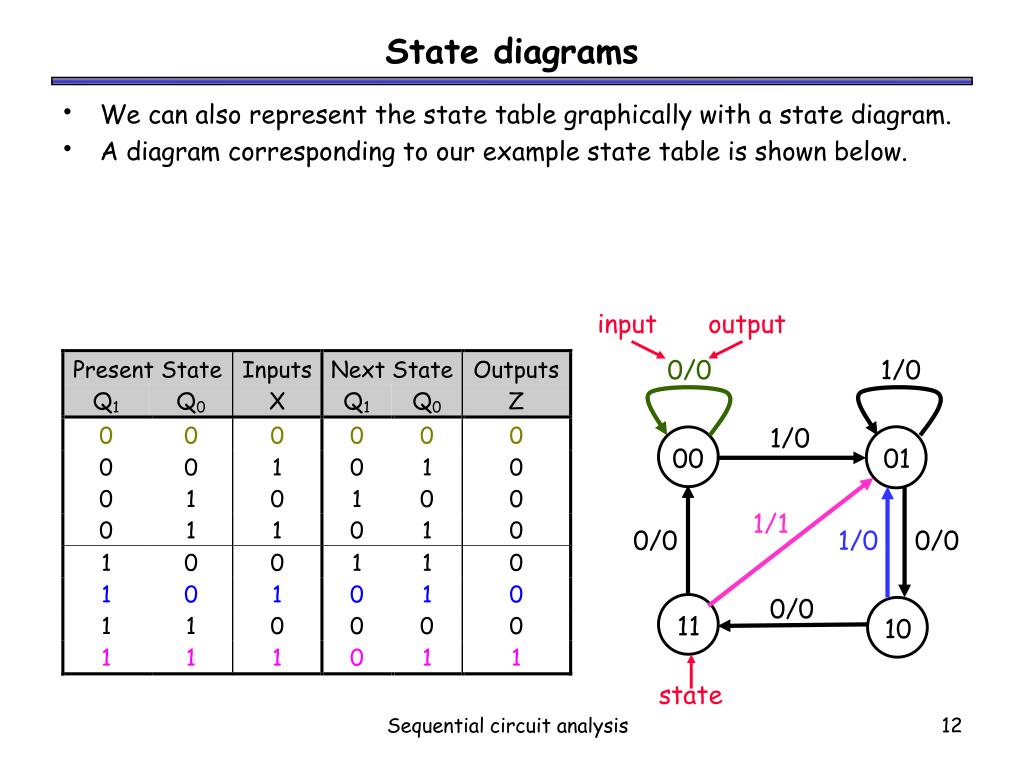
Our new tool can produce TSA analyses/plots for both raw and estimated binomial summary measures that are above and beyond what was available previously, and overcomes the limitations in the TSA-software that is currently available. Results: using data from a published systematic review, Figure 1-2 shows the results of the validation analyses. A TSA plot is generated for cumulative Z-scores on the y-axis and cumulative information size from trials on the x-axis, which compares these Z-scores with the monitoring and futility boundaries (Figure 1). To conduct TSA we need a predefined baseline risk, relative risk reduction, type I and type II error probabilities, and either a predefined - or estimated - between-study heterogeneity from the available data.
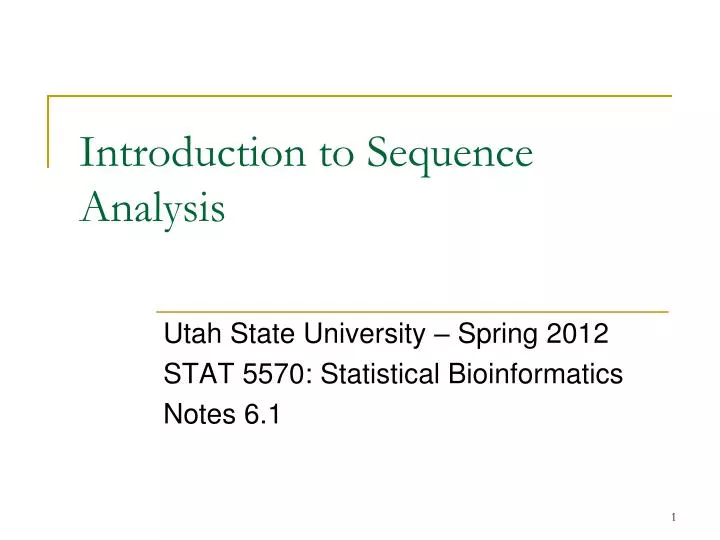
This tool allows for calculation of the required information size (RIS)/sample size, monitoring boundaries for type I errors using the alpha spending function, and conditional power boundaries for futility using a beta spending function, with a predefined conditional bound gamma. Methods: our TSA tool is programmed in the open-source R language building on the 'tidyverse' package (required for the R function) and 'shiny' (required to run the web-based application). Objectives: the goal of this study is to describe the development and testing of a user-friendly tool to conduct TSA for both raw and summary effect measures. In order to increase its uptake and usability, we implemented the TSA methods in the open-source R environment, and developed a user-friendly web-based application for binomial raw and summary effect measures. TSA is a natural bridge between cumulative meta-analysis and sequential analysis of clinical trials, and offers methods for dealing with repeated testing bias, heterogeneity among studies, and measuring significance and futility precisely.
#SEQUENTIAL ANALYSIS TRIAL#
To overcome this problem, trial sequential analysis (TSA) accounts for bias and observed heterogeneity in a cumulative meta-analysis. However, meta-analyses introduce problems inherent to multiple testing as new trials are added over time. The basic principles of sequential analysis consist of the following.Background: meta-analyses increase both the power and the precision of estimated treatment effects. He established that in the problem of decision (based on the results of independent observations) between two simple hypotheses the so-called sequential probability ratio test gives a considerable improvement in terms of the average number of observations required in comparison with the most-powerful test for deciding between two hypotheses (determined by the Neyman–Pearson lemma) for a fixed sample size and the same error probabilities. The intensive development and application of sequential methods in statistics was due to the work of A. Its characteristic feature is that the number of observations to be performed (the moment of termination of the observations) is not fixed in advance but is chosen in the course of the experiment depending upon the results that are obtained. 2010 Mathematics Subject Classification: Primary: 62L10 Ī branch of mathematical statistics.
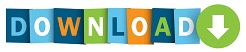